Keynotes
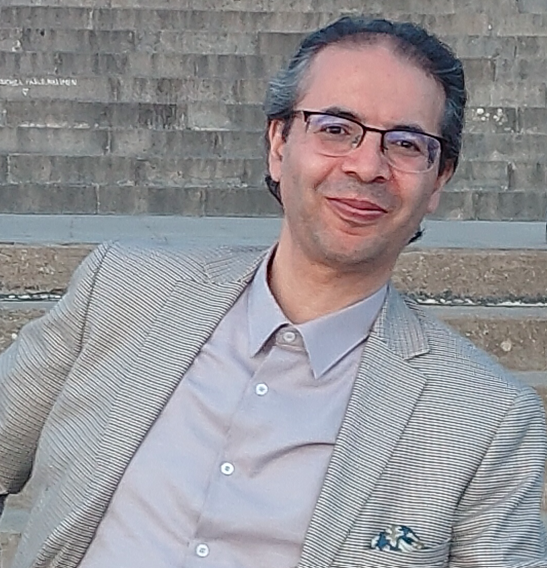
Speaker: Prof. Saïd Mahmoudi
Affiliate: University of Mons, Belgium
Keynote title: "Computer-aided diagnosis with Federated Learning: the case of breast cancer screening"
Saïd Mahmoudi: received his MS in Computer Science from University of Lille1, France in 1999. He obtained his PhD in at the University of Lille1, France in 2003. Between 2003 and 2005, he was Associate Lecturer at the University of Lille3, France. Since September 2005, he is Professor at the Faculty of Engineering of the University of Mons, Belgium. His research interests include artificial intelligence, medical images analysis, internet of things, , 2D and 3D retrieval and indexing, Big Data and large-scale multimedia management. He published over then 200 journal and conference papers to date. He participates in numerous scientific and academic activities (member of the organizing committee for some conferences, reviewer and guest editor for several internationals journals). Prof. Saïd MAHMOUDI was involved in several projects including industrial and academic researchers, in an international context. He is editor of some journals like Nature Scientific reports and Springer Multimedia Tools and Applications.
Abstract: Federated learning is a new learning paradigm dedicated to preserve data privacy by achieving collaborative learning including several devices or centers without moving the data from their original location. This new learning method aims to create a global shared model form a set of decentralized datasets. It allows a global model to learn from several distributed sub-models, improving overall performance without compromising individual subsets. Within this paradigm, individual participants do not exchange their local data, but local centers train similar model on their own data. The use of Federated Learning for breast cancer early diagnosis and analysis will be discussed. On the other hand, automated labelling, and localization of mammographic masses and microcalcifications will be presented. Also, there are some security issues that must be tackled when using Federated Learning paradigm. These issues will be presented and discussed. The existing countermeasures studied in the literature like differential protection, secure multi-party computation, etc. will be presented.
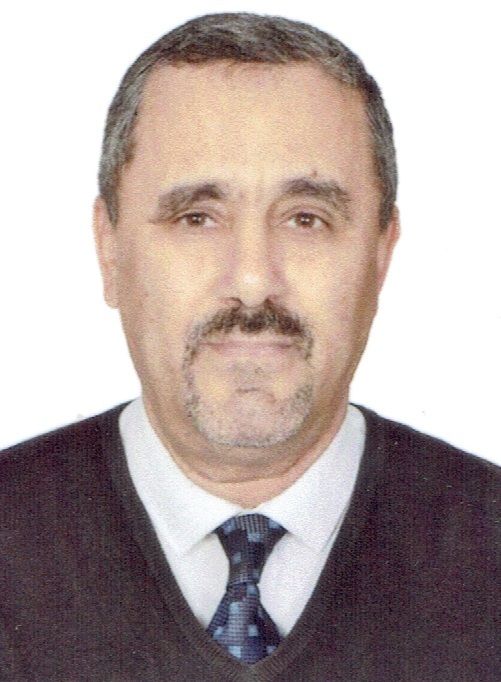
Speaker: Prof. Mounir Sayadi
Affiliate: Tunis University
Keynote title: "Textures images modeling and characterization: Methods and applications"
Mounir Sayadi: is Professor of signal and image processing at the National Superior School of Engineers of Tunis (ENSIT), Tunis University, and Head of Laboratory of Signal Image and Energy Mastery (SIME). He received the BSc degree in Electrical Engineering from the High School of Sciences and Techniques of Tunis, the DEA degree in automatic and signal processing from same school and the PhD degree in signal processing from the National School of Engineers of Tunis, respectively in 1992, 1994 and 1998.
Prof. Sayadi has published over 60 research journal papers and more than 150 scholarly communications in many international conferences. Prof. Sayadi has supervised 16 PhD and 45 Master in the field of computer science, signal and image processing. He serves as a referee for many international journals and he is a organizing member of several international conferences and research projects. His research interests are focused on signal, image processing and filtering and bio-medical data analysis with computational intelligence.
Abstract: The texture analysis plays a significant role in many image processing and pattern recognition applications such as remote sensing, cartography, document analysis, robot vision, military surveillance, environment modeling, content-based image retrieval, industrial and food product quality control and medical imaging. Many approaches for texture characterization have been developed for modeling, analysis, segmentation and synthesis. The traditional texture characterization is based on extracting some statistic features from the pixel domain data (using histograms, autocorrelation, and moments). The co-occurrence matrix is also a popular statistical technique for extracting textural features. Furthermore, the texture image can be modeled as a Markov random field of pixels level, where the relationships between the gray levels of neighboring pixels are statistically characterized. In spite of this, feature-based approaches have been also proposed which is sometimes less computationally demanding and more efficient. Textural features can be extracted by using spectral information, Wavelet basis functions and 2-D Gabor filters. In addition, the Local Binary Pattern (LBP) method has also been proposed for texture characterization. More recently, an approach for texture characterization and synthesis based on Textons (texture elements) was proposed where textures are assumed to be composed of three components, namely illumination, structure, and stochastic. Also, a unified texture model based on 2-D Wold-like decomposition was proposed. This model is appropriate for modeling real-world textures and describing its randomness. The texture is decomposed into three mutually orthogonal components: stochastic, harmonic and generalized-evanescent component. One part of the 2-D Wold model of gray-scale textures is the 2-D stochastic component. This component can be represented by a 2-D Auto-Regressive parametric model, commonly used in signal processing, which offers an expression of the spatial interaction among the neighbors pixel in the image. The parametric model (using transversal and reflection coefficients) can be estimated by using existing adaptive estimation methods of purely stochastic random fields. The linear adaptive parametric filters fail in some applications, such as image enhancement, modeling and compression because of the nonlinear and non-stationary usual character of images. Furthermore, experimental results indicate that some processing levels of the visual system have nonlinear characteristics. Looking for better results, nonlinear adaptive filtering i.e. adaptive 2-D Volterra filters and 2-D AR exponential filter, has been proposed. To evaluate how much models improves the texture classification, two characterization criteria are conventionally used in the pattern recognition field. The first is a classification accuracy based on a weighted Euclidean distance classifier. The second is a characterization degree based on the ratio between the "inter-variance" and the "intra-variance" of the coefficients. The greater the characterization degree is, the more robust the classification process is. Finally, for texture segmentation applications, we can extract a feature vector from a sliding window and use the Fuzzy C-means clustering technique to cluster the image into several classes corresponding to the different texture of the image.
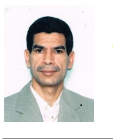
Speakers: Prof. Mohammed Said Souid and Dr. Sabit Souhila
Affiliate: Tiaret University , Algeria
Keynote title: "The calculation of variable order fractional derivatives and numerical computation"
Mohammed Said Souid: is a Professor Doctor of mathematics at the University of Tiaret, Algeria. He holds a magister in 2011 and doctorate degrees in sciences, mathematics in 2015 from Sidi bel Abbas University, Algeria. Enseignant at the Faculty of Economics, Comercial and Management Sciences at Ibn Khaldoun University, Tiaret, since November 2013. His main researches include fractional differential equations and inclusions.
Sabit Souhila: is an associate Professor Doctor of mathematics at the University of Tiaret, Algeria. She holds a magister in 2012 in ENS-Kouba Alger and doctorate degrees in sciences, mathematics in 2014 from Rennes University, France. Enseignant at the Faculty of Mathematics and Informatics at Ibn Khaldoun University, Tiaret, since October 2014. Her main researches include Numerical Methods for Reactive Transport in porous media, Partial Differential Equations and now a Numerical Methods for fractional differential equations.
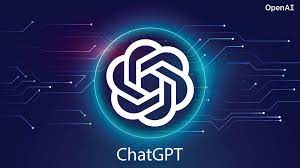
Speakers: Dr. Mohamed Goudjil and Dr. Belhacene Bahloul.
Affiliate: Khemis Miliana University , Algeria
Keynote title: "From Data to Actionable Insights: Harnessing NLP and ChatGPT to Drive Business Intelligence Success"
Mohamed Goudjil: is a highly accomplished computer engineering expert with extensive experience in the field of machine learning and artificial intelligence. He work as an Ass.Professor at Khemis Miliana University. Prior to this, Goudjil worked at various establishments, including the Advanced Technologies Development Centre (Microelectronic Division) and AL-JOUF University in the Kingdom of Saudi Arabia, where he taught courses such as Microprocessor Systems, FPGA, Digital Design, and Programming Languages. Throughout his career, Goudjil has conducted research in the field of machine learning, with a particular focus on text categorization using active learning techniques and copula functions. He has also published several articles in reputable journals, on topics such as Active learning, Arabic text categorization and text summarization. Additionally, Goudjil has participated in numerous academic committees, including the Self-Study Committee of Computer Engineering Program and the Committee of Quality & Academic Accreditation.
Abstract:In today's data-driven world, businesses face the formidable task of extracting actionable insights from the immense volume of information at their disposal. The advent of Natural Language Processing (NLP) and advanced language models like ChatGPT has revolutionized the field of business intelligence, empowering organizations to convert raw data into valuable insights. NLP techniques enable the analysis of unstructured data sources such as customer reviews, emails, and social media posts, automating text analysis, sentiment analysis, and topic modeling. ChatGPT, a state-of-the-art language model, adds conversational capabilities to the business intelligence process, engaging in interactive conversations, generating reports, and providing real-time insights. Integration of NLP and ChatGPT into business intelligence platforms equips decision-makers with the tools to identify emerging trends, understand customer preferences, optimize operations, and develop effective marketing strategies. However, challenges exist, including ensuring data quality, interpretability of results, bias mitigation, and privacy protection. Responsible and ethical use of NLP and ChatGPT empowers businesses to gain a competitive edge by making informed data-driven decisions. These technologies unlock the potential to extract valuable insights from unstructured data, enhancing customer understanding, and driving organizational growth.
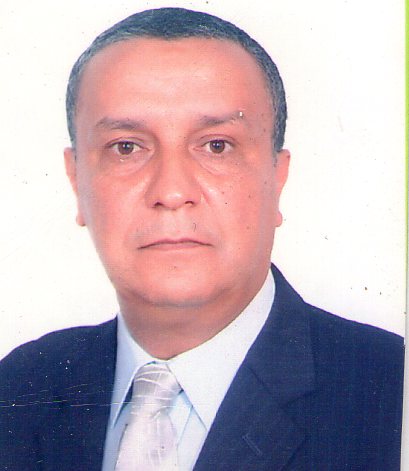
Speaker: Pr.Abou El Hassan BENYAMINA
Affiliate: University of ORAN1 Ahmed Ben Bella , Algeria
Keynote title: "L’agriculture intelligente & La sécurité alimentaire : retour d'expérience sur des projets nationaux et internationaux"
Abou El Hassan BENYAMINA: is a Full Professor Computer Science Department University of ORAN1 Ahmed Ben Bella ALGERIA. Director of LAPECI Laboratory. Coordinator Prima WaterMed4.0 Project.
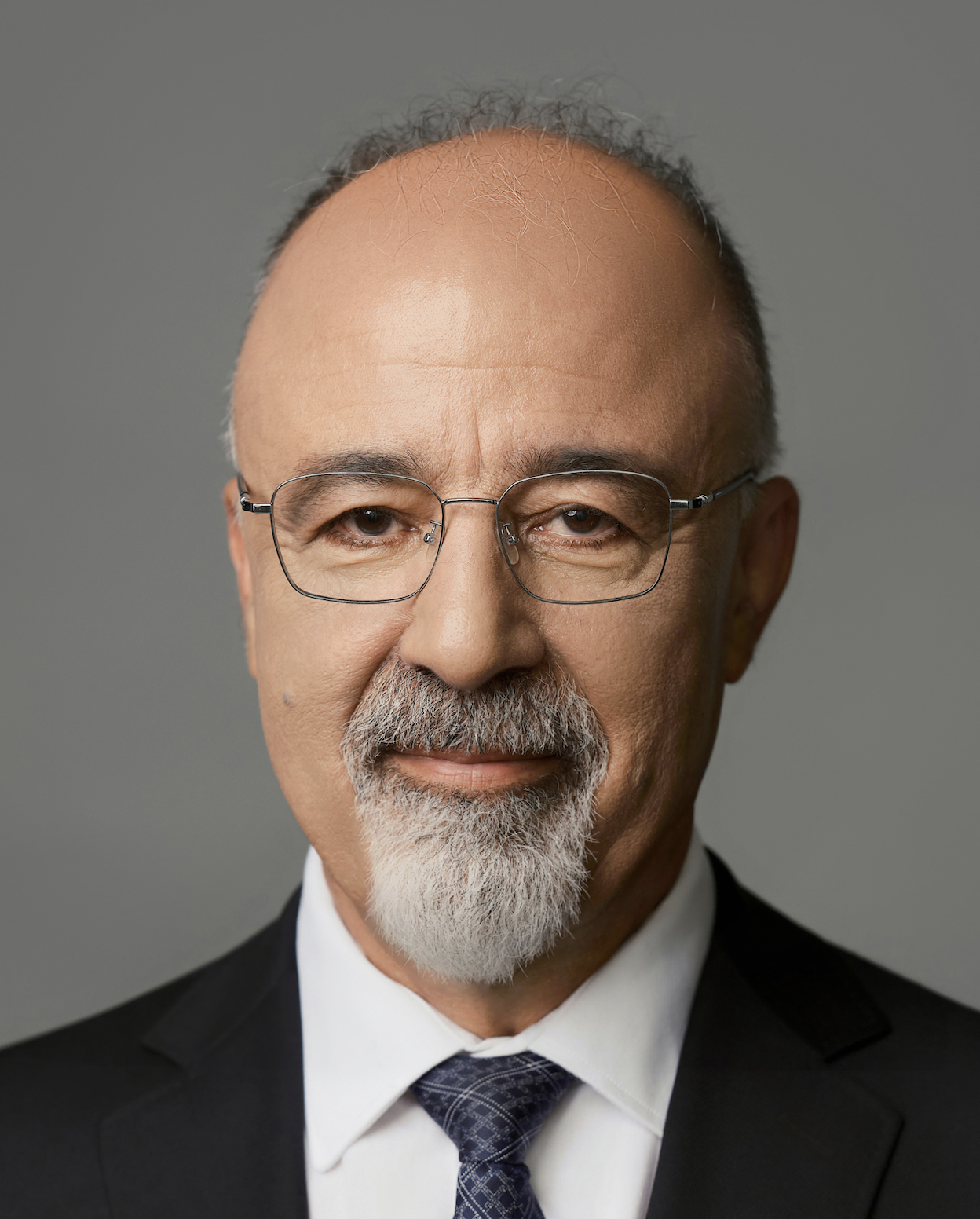
Speaker: Pr.Mohamad Sawan
Affiliate: Westlake University, Hangzhou, China
Keynote title: "Biosensors to Screen Neural Activities Belonging to Brain Disorders"
Mohamad Sawan: Mohamad Sawan is Chair Professor in Westlake University, Hangzhou, China, and Emeritus Professor in Polytechnique Montreal, Canada. He is founder and director of the Center of Excellence in Biomedical Research on Advanced Integrated-on-chips Neurotechnologies (CenBRAIN Neurotech) in Westlake University, Hangzhou, China. Also, he is founder of the Polystim Neurotech Laboratory in Polytechnique Montréal. He received the Ph.D. degree from University of Sherbrooke, Canada. Dr. Sawan research activities are bridging micro/nano electronics with biomedical engineering to introduce smart medical devices dedicated to improving the quality of human life. He is co-founder and was Editor-in-Chief of the IEEE Transactions on Biomedical Circuits and Systems (2016-2019). He hosted the 2016 IEEE International Symposium on Circuits and Systems, and the 2020 IEEE International Medicine, Biology and Engineering Conference (EMBC). He was a Canada Research Chair in Smart Medical Devices (2001-2015), and was leading the Microsystems Strategic Alliance of Quebec, Canada (1999-2018). Dr. Sawan published more than 1000 peer reviewed papers and many books and patents. Among the numerous received honors, Dr. Sawan received the Chinese National Friendship Award, the Lebanese’s President Medal of Merit, the Shanghai International Collaboration Award, the Queen Elizabeth II Golden Jubilee Medal. Dr. Sawan is Fellow of the Royal Society of Canada, Fellow of the Canadian Academy of Engineering, Fellow of the IEEE, and “Officer” of the National Order of Quebec.
Abstract: Nowadays, emerging neurotechnology is spread to implement smart medical biosystems (sensors and actuators) intended to enhance or recover lost vital functions, mainly due to brain disorders. To meet such needed efficient biodevices, smart biosensors to screen neural activities, and monitor and manipulate cells and study neural diseases, and links with brain diseases. Also, learning algorithms and corresponding neuromorphic hardware are becoming the main option of emerging neural network-based microchips to introduce needs tools for this above introduce purpose. This talk covers wearable and implantable microdevices based on custom system-on-chip (SoC) integrated platforms. These biosensors and actuators deal with multidimensional design challenges such as power management, low-power and high-data rate wireless communication methods, intended to implement brain interfaces. Case studies include various neurorecording types for several applications such as epilepsy, stroke, addictions, and multi-virus detectors.